Managing QoE under disruptive conditions
Previously published on Telecoms.com
Author: Scott Sumner
May 18, 2020
Telecoms.com periodically invites expert third parties to share their views on the industry’s most pressing issues. In this piece Scott Sumner, Director of Commercial Insight at EXFO, looks at the challenges of ensuring quality of experience in the current unique circumstances.
During times of crisis—pandemics, conflict, natural disasters—service providers face challenging conditions at a time when their customers are heavily dependent on telecom services. Abnormal, dynamic traffic patterns trip off seas of alerts.
When subscribers are forced into new routines, traffic volumes spike in locations and at times that would otherwise be relatively quiet. The population turns to social media, voice and video calls to check on loved ones and establish response plans with their employers. Remote working and streaming video consumption drive high utilization in residential areas.
While users may understand that networks are facing unprecedented demand, telecom brands will be punished if they fail to deliver consistent performance.
Net promotor score (NPS)—a key metric closely tied to churn, customer lifetime value (CLV) and profitability—is 49% influenced by mobile customers’ network experience. When quality of experience (QoE) is not perceived to be ‘very good’, promotors become detractors.
Telecom brands can be quickly damaged, but not quickly repaired: when subscribers leave their provider they will not return for at least five years. Retention costs rise, and margins erode. [sources: IPSOS, and Portevo, 2019]
Rising complexity
Addressing service degradation and outages is hard when operations staff are working remotely, and deafening alarm noise impedes their ability to isolate fault origins. Maintaining peak performance under these conditions strains existing processes that are largely manual and serial in nature.
Consider a recent survey by Heavy Reading that showed operations teams typically use six service assurance tools and require 12 experts from three different domains to resolve even minor outages. Lots of emails, SWAT teams, spreadsheets… it feels like wartime conditions even under normal circumstances.
Current crisis conditions foreshadow the traffic patterns that will be introduced by highly dynamic 5G services and the new enterprise and IoT applications they will support. The GSMA notes that by 2025 there will be five times more machine ‘mobile subscribers’ than humans, and network traffic growth is projected at 53% per year over the same period. We won’t be returning to the same normal we’re used to, no matter how we manage our way out of the current pandemic.
With an increasing degree of virtualization and orchestration adding to the mix, the complexity operations teams face is beginning to exceed their resources—both tools and staff. So, the vast majority of service providers are turning to automation and machine learning approaches to prepare for a continued state of ‘abnormal’. This strategy also gives their scarce experts superpowers to reveal and resolve issues proactively, even prescriptively.
Refocusing efforts
One key lesson learned is that the focus of operations teams needs to change. Instead of alarms, they need to focus on customer-impacting events. At first glance, this may appear similar: lots of alarms should indicate a fault affecting many customers. But there is often a shaky correlation between alarms and quality of experience.
Consider a cell tower that loses power from the grid. A series of alarms will kick off. Is this a major problem? It’s hard to tell. Cells are made to be redundant; neighbors can often pick up subscribers. Maybe the cell is still working fine on a generator. Maybe it’s totally down, but at a location or time of day where there are few people even using it.
This simple example illustrates why operations teams are learning they need to prioritize action based on the actual number of customers impacted, not simply network faults. It also means they need to detect degradations. These short term ‘silent failures’ are significantly more impactful to customers trying to ‘get things done’ than the rare outage.
In a recent incident at a North American mobile operator, a significant number of customers were unable to place or receive calls. What started as a small service issue rapidly ramped into a large-scale problem—but remained undetected by the operator for nearly three hours.
This is typical to degradations that occur under high utilization conditions. The issue affected a relatively small density of customers across a large geographic region—resulting in individual experiences that were effectively invisible to monitoring systems.
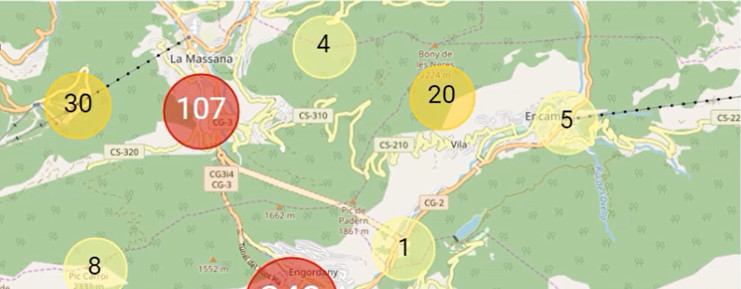
Under highly dynamic traffic conditions, customer-impacting events can suddenly appear and rapidly escalate, often as the result of overloaded core network functions (e.g. authentication servers, scalability of vIMS functions).
This requires a new way of detecting, assessing and resolving customer-impacting events quickly, and directly—not inferred from network metrics—before they escalate into large scale outages.
It’s a difficult problem for traditional big data analytics. Certainly, this is a big data problem, but the approach of storing metrics and data feeds then looking for user experience issues is compute- and time-intensive.
Real-time analytics – reveal the invisible
Machine learning methods that use stream-processing platforms like Apache’s Spark are designed to overcome these limitations by analyzing data as it arrives, in real-time. This is where innovation is centering on a new breed of customer experience analytics. The wealth of available open-source analytics and machine learning platforms are redefining what is possible, and scalable.
Operations teams are now experimenting with real-time detection tools that use machine learning to detect QoE issues, assess the impact, and diagnose the root cause within minutes. This permits operations to see which issues are most important to address, and what action to take—without sifting through alarms or consulting multiple monitoring systems.
Machine learning is allowing them to see through a new lens that derives significantly more insight from existing systems. This allows operations teams to resolve issues faster and deliver an excellent quality of experience that drives loyalty, subscriber count and margin in challenging times. When things will unlikely ever be ‘normal’ again, it’s good that there are new tools emerging that will help us remain in control.